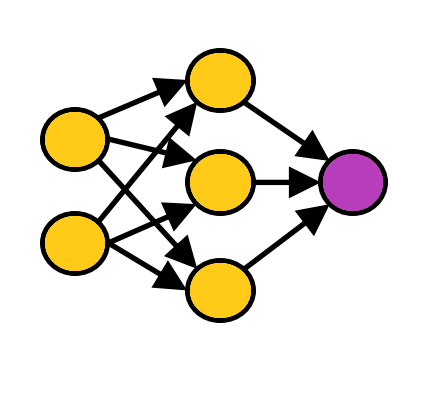
LEARNING OUTCOMES
Students can formalize applications as ML problems and solve them using basic ML methods.
Students can perform basic exploratory data analysis.
Students understand the meaning of the train-validate-test approach in machine learning.
Students can apply standard regression and classification models on a given data set.
Students can apply simple clustering and dimensionality reduction techniques on a given data set.
Students are familiar with and can explain the basic concepts of reinforcement learning.
Credits: 5
Schedule: 04.09.2023 - 13.10.2023
Teacher in charge (valid for whole curriculum period):
Teacher in charge (applies in this implementation): Pekka Marttinen, Stephan Sigg
Contact information for the course (applies in this implementation):
CEFR level (valid for whole curriculum period):
Language of instruction and studies (applies in this implementation):
Teaching language: English. Languages of study attainment: English
CONTENT, ASSESSMENT AND WORKLOAD
Content
valid for whole curriculum period:
Exploratory data analysis.
Dimensionality reduction, PCA.
Regression and classification.
Clustering.
Deep learning.
Reinforcement learning.
Assessment Methods and Criteria
valid for whole curriculum period:
Assignments, project report, participation in peer-grading.
Workload
valid for whole curriculum period:
5 credits approx. 134 hours of work divided into
Lectures + self-study: 10*(2+3)=50 hours
Assignments: 6 * 9 = 54 hours
Project work: 26 hours
Peer-grading: 4 hours
DETAILS
Substitutes for Courses
valid for whole curriculum period:
Prerequisites
valid for whole curriculum period:
FURTHER INFORMATION
Further Information
valid for whole curriculum period:
Teaching Language : English
Teaching Period : 2022-2023 Autumn I
2023-2024 Autumn I