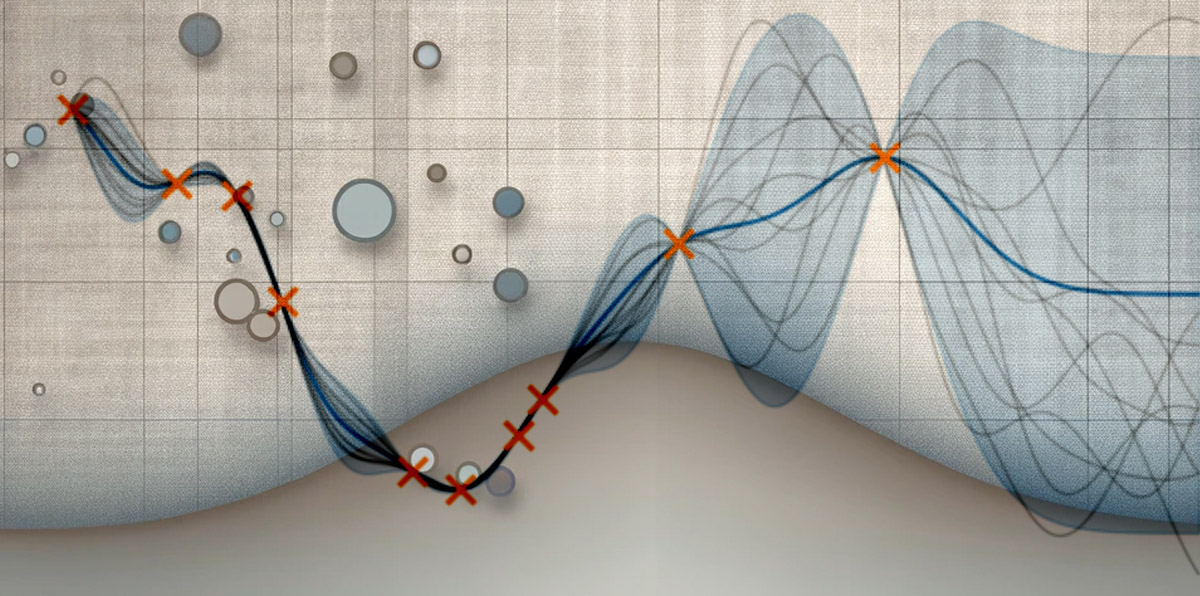
LEARNING OUTCOMES
The course gives a comprehensive overview of Gaussian processes (GPs) in machine learning. After the course, the student can recognize where GPs can be used and know their limitations as well as their theoretical underpinnings. The student is familiar with the most common approximative inference methods for GPs and is familiar with their contemporary use and applications.
Credits: 5
Schedule: 24.02.2025 - 03.04.2025
Teacher in charge (valid for whole curriculum period):
Teacher in charge (applies in this implementation): Arno Solin, Ti John
Contact information for the course (applies in this implementation):
CEFR level (valid for whole curriculum period):
Language of instruction and studies (applies in this implementation):
Teaching language: English. Languages of study attainment: English
CONTENT, ASSESSMENT AND WORKLOAD
Content
valid for whole curriculum period:
Gaussian processes (GPs) are a powerful machine learning paradigm for Bayesian nonparametric modelling. This course will give an overview of Gaussian processes in machine learning, and it provides both a theoretical and practical background for leveraging them. The course covers Gaussian process regression, classification, and unsupervised modelling, as well as a selection of more recent specialised topics. This is a more specialized course and assumes students already have a background in machine learning.
Assessment Methods and Criteria
valid for whole curriculum period:
Solving/returning assignments and/or a final exam.
Workload
valid for whole curriculum period:
Contact teaching c. 36 h, assignments related to course content c. 40 h, independent work c. 60 h.
DETAILS
Study Material
valid for whole curriculum period:
Lecture notes and other given material.
Substitutes for Courses
valid for whole curriculum period:
Prerequisites
valid for whole curriculum period:
SDG: Sustainable Development Goals
9 Industry, Innovation and Infrastructure
FURTHER INFORMATION
Further Information
valid for whole curriculum period:
Teaching Language: English
Teaching Period: 2024-2025 Spring IV - V
2025-2026 Spring IV - V